About us
Transforming Women's Health with AI Ultrasound
At Scanvio Medical, we improve health by making expert ultrasound available to everyone. We help millions of women get a faster endometriosis diagnosis with our AI-augmented ultrasound software. Our solution provides every gynecologist with expert sonography skills.
We are an ETH Zurich Spin-off, founded in 2024. Our team members have extensive experience in gynecology, machine learning, and building successful solutions for medical technology and surgical education.
We are an ETH Zurich Spin-off, founded in 2024. Our team members have extensive experience in gynecology, machine learning, and building successful solutions for medical technology and surgical education.
Our Partners

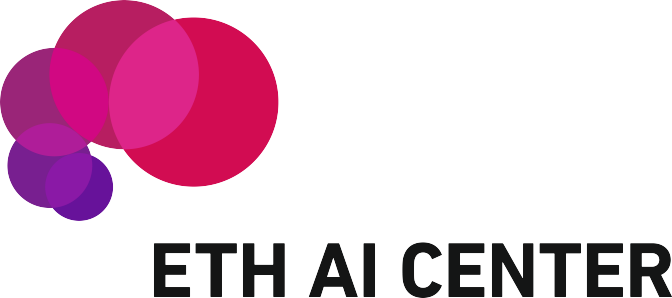
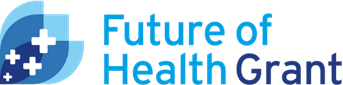
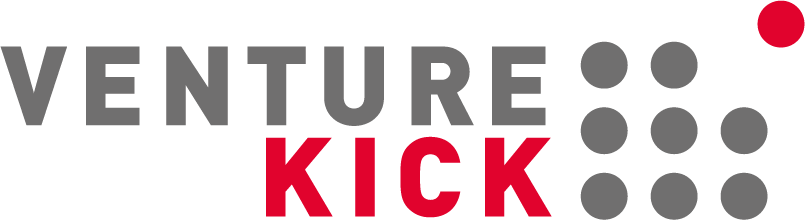
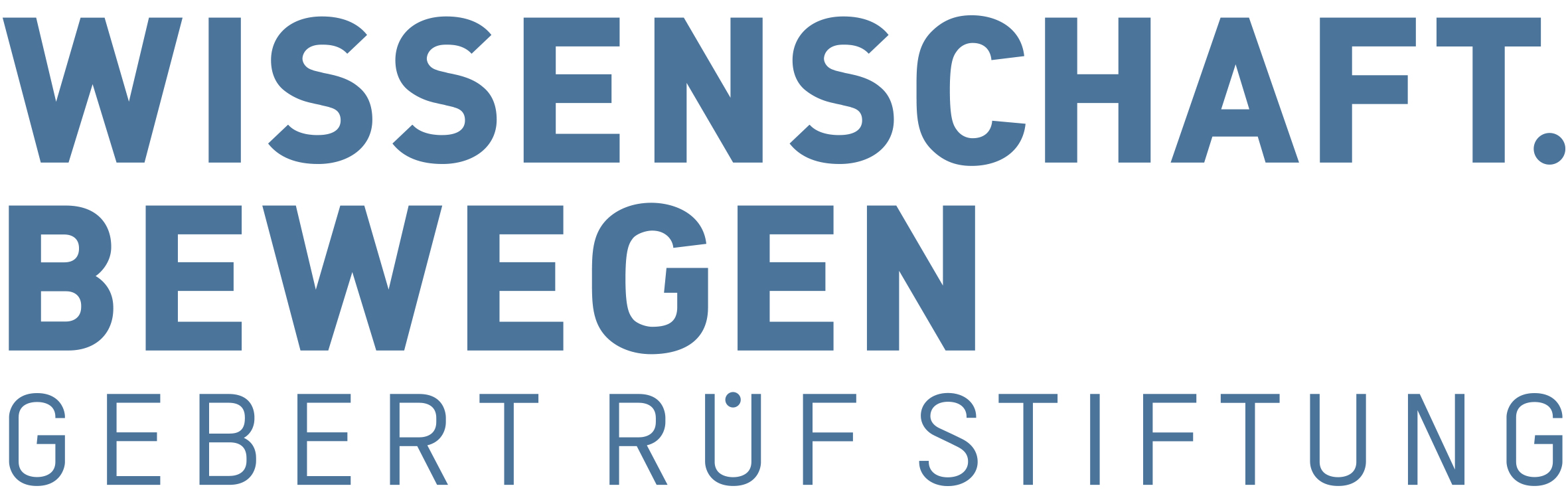

Join us!
Meet our team
Our team specializes in gynecology, machine learning, and developing medical technology and surgical education solutions.
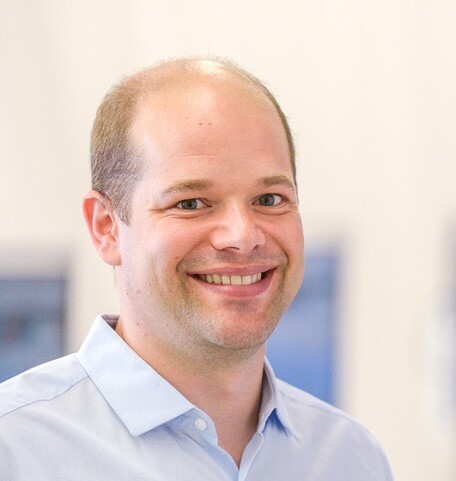
Stefan Tuchschmid, Ph.D.
Chief Executive Officer
Stefan shaped his ETH Zurich PhD thesis into VirtaMed, a global leader in surgical training with 130+ employees. He's a winner of the Swiss Economic Award in Hightech/Biotech and a serial winner of most business plan competitions in Switzerland. Before starting Scanvio Medical, he mentored aspiring student entrepreneurs and start-up / scale up companies. Stefan supports strategic research at the ETH AI Center within surgery, medtech and health-related activities.
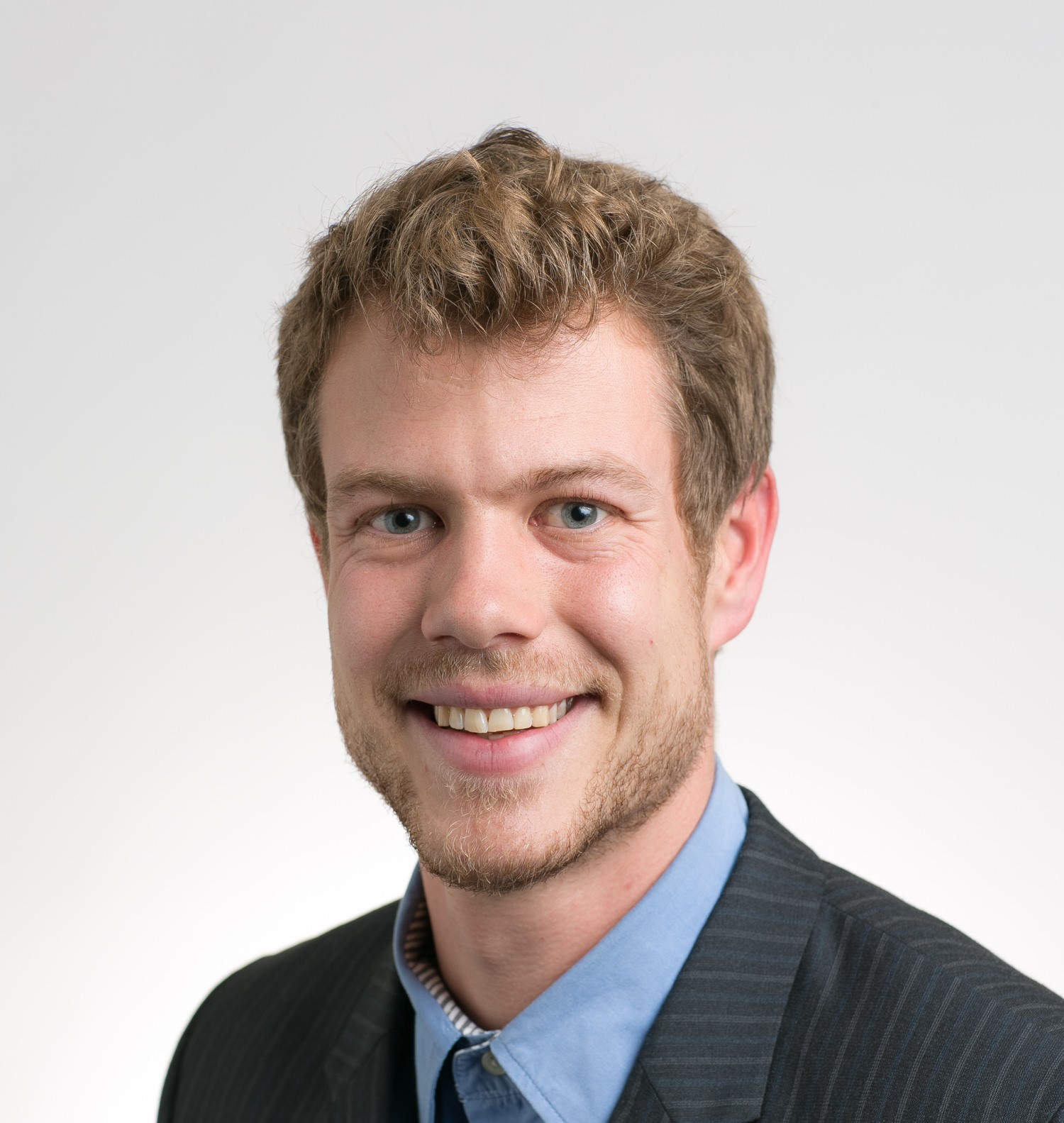
Fabian Laumer, Ph.D.
Chief Technology Officer
Fabian finished his PhD Thesis entitled “Deep Learning for 3D Heart Shape Reconstruction in Echocardiography” at the Institute for Machine Learning at ETH Zürich in 2023. His research focused around the development of intelligent algorithms for automated and robust interpretation of ultrasound videos. In 2021 Fabian Laumer received the ML4H NeurIPS2020 best paper award.

Michael Bajka, M.D., Prof.
Chief Medical Officer
Michael is a specialist in obstetrics and gynecology with educational responsibility, particularly in surgery, ultrasound, and endometriosis. Since 1994, Michael Bajka has been a national leader in gynecologic ultrasound while pursuing a passionate career as a clinical practitioner. His central interests are basic research, prototyping, clinical validation, establishing curricula, and assessing future surgeons and ultrasound specialists. He established national and international contacts between medical experts of different specialties, leading to a broad network in all aspects of ultrasound.
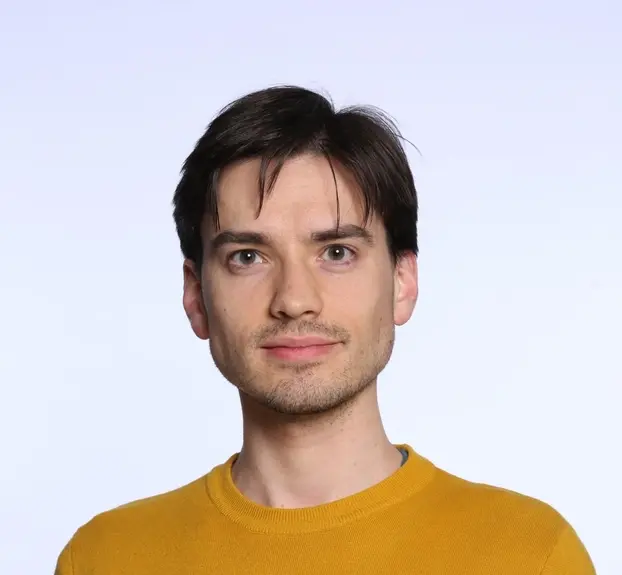
Gabriel Fringeli, M.Sc. ETH Zürich
Lead AI Engineer
Gabriel Fringeli earned his Master and Bachelor degree in Computer Science from ETH Zurich. His focus of study was on machine learning with applications in the areas of medicine and software security and includes an awarded publication at NeurIPS 2020 on information extraction for cardiac ultrasound data. Before joining Scanvio, Gabriel worked as a software and AI engineer at Snyk developing machine learning methods for fixing source code vulnerabilities.
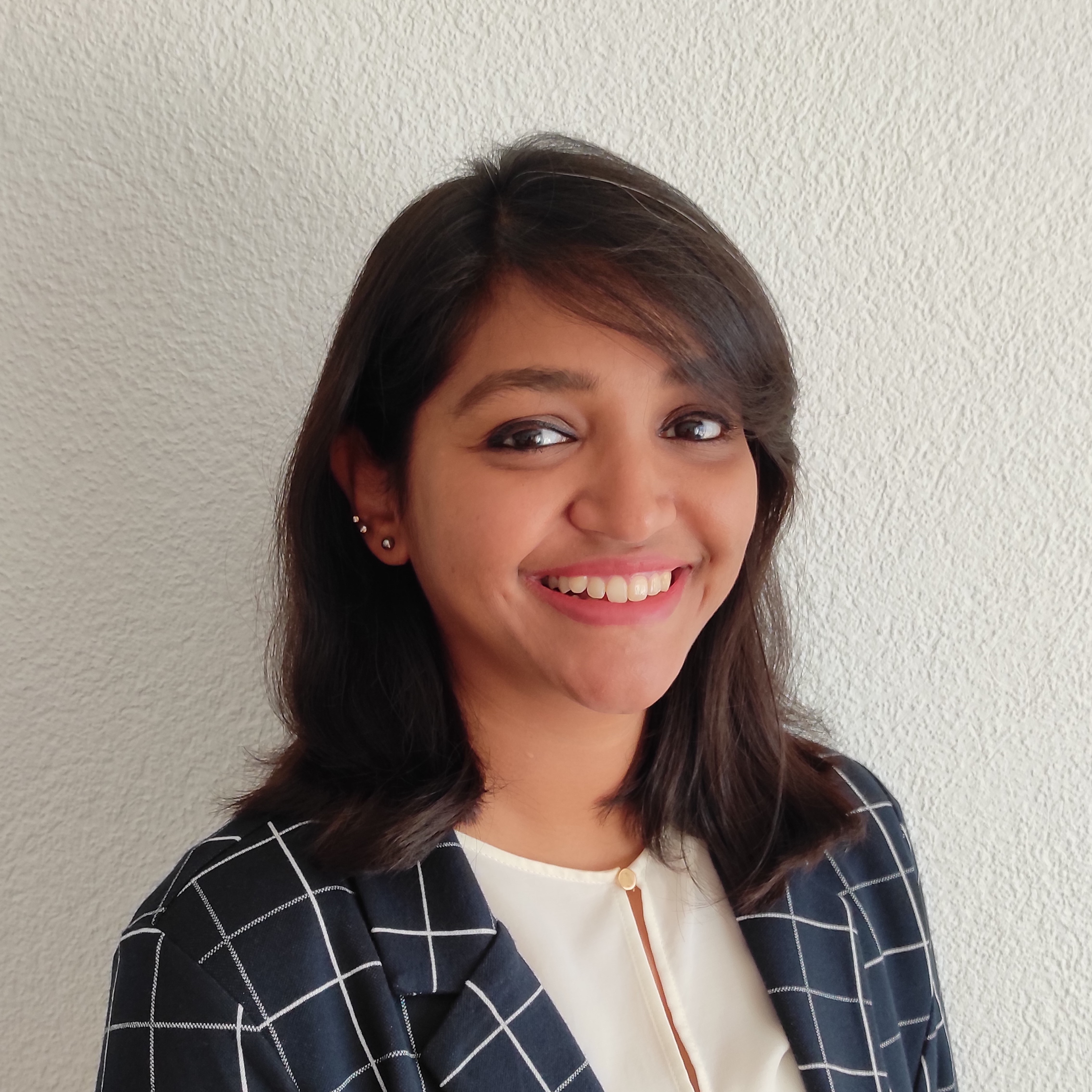
Vaishnavi Shriram, MURP, Student - M.Sc. Business Administration, HSLU
Founder's Associate
Vaishnavi is an experienced and driven professional currently pursuing an M.Sc. in Business Innovation and Entrepreneurship, with a strong drive to support value-driven startups that prioritize societal impact. She brings a comprehensive skill set in strategic planning, digital marketing, and project management to her role at Scanvio Medical. Having worked in research and consulting, Vaishnavi has extensive experience in data analytics, market research, business development, and client engagement.

Sergio Tascon-Morales, Ph.D.
Senior Machine Learning Engineer (Innosuisse Project with ETH)
Dr. Sergio Tascon-Morales completed the Erasmus Mundus Joint Masters in Medical Imaging and Applications (MAIA) in 2020, receiving the titles of best student and best thesis. During his Ph.D. at the University of Bern, he carried out research in the field of Visual Question Answering (VQA), achieving publications at top-tier conferences like CVPR and MICCAI. His research interests include computer vision and natural language processing, as well as multimodal learning applied to medical data.

Claudia Berger, MPA
Clinical Data Coordinator
With over 20 years of experience in the medical field, Claudia brings extensive knowledge and expertise to the Scanvio team. Her background as a medical assistant, combined with her training as a chemical laboratory technician and chemist, equips her with a strong scientific foundation and a structured approach to her work. At Scanvio, Claudia is responsible for the meticulous processing of medical data, ensuring accuracy and reliability in her daily tasks.
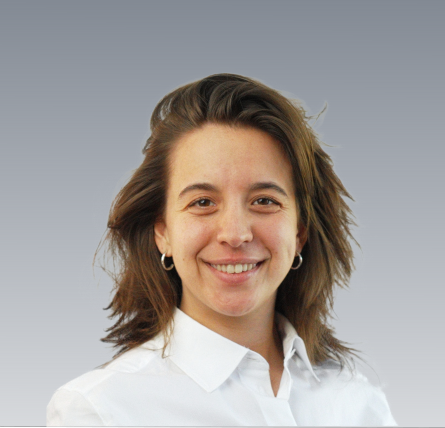
Lavinia Schlyter, M.Sc. Computational Science, EPFL
AI Software Engineer
Lavinia holds a Master’s degree in Computational Science from EPFL, where she focused on data analysis, numerical simulations, and advanced machine learning techniques. After completing her master’s thesis at ETH Zurich, she extended her work as a research assistant in the Computer Vision Lab within the Biomedical Imaging Group. Her research was focused on anomaly detection in the blood flow of the aorta using 4D flow MRI scans, leveraging self-supervised deep learning methods with creating and incorporating synthetic anomalies.
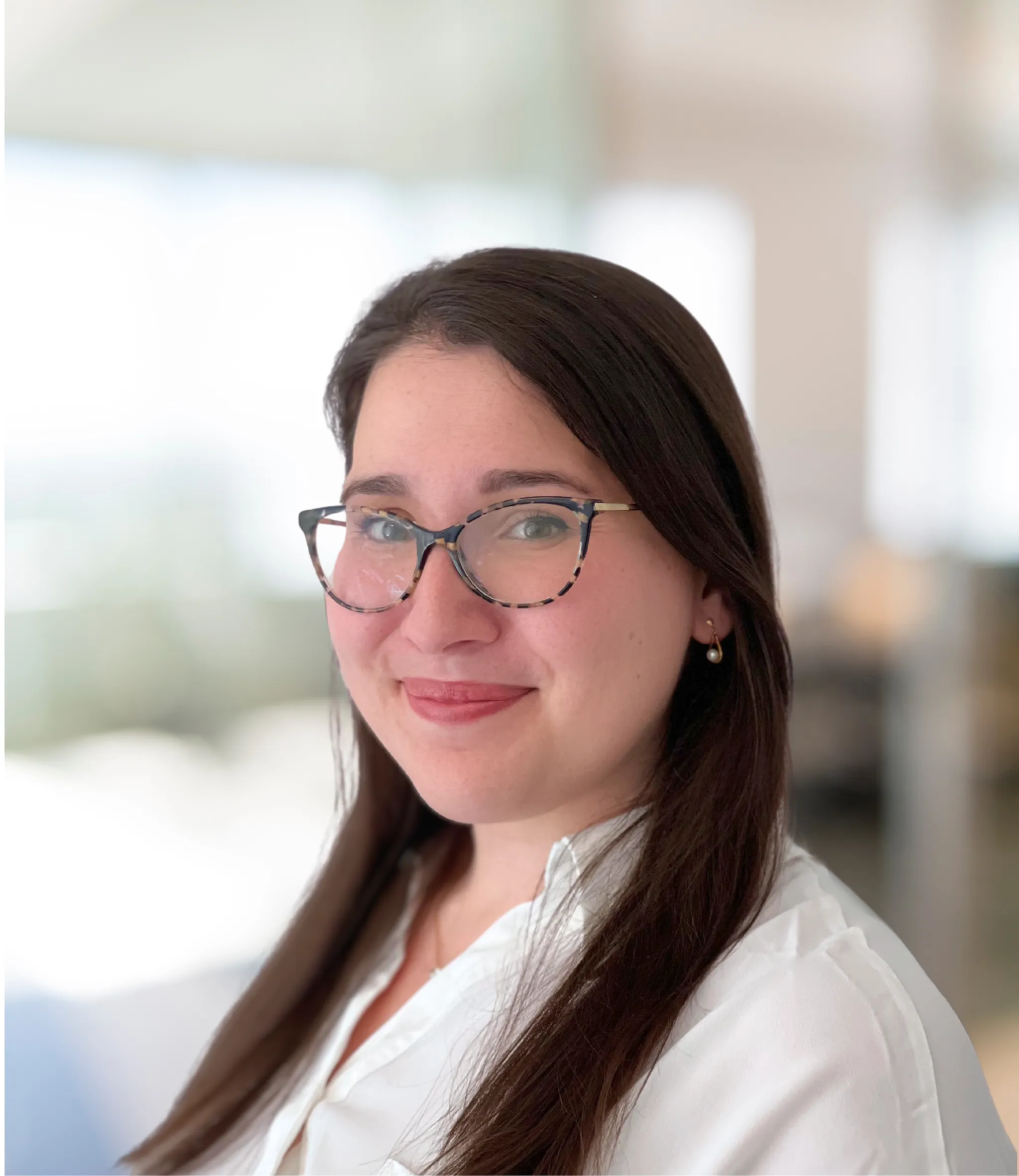
Maria Narciso, Ph.D.
Clinical Operations Manager
Dr. Maria Narciso earned her PhD in Medicine and Translational Science from the University of Barcelona through the Marie Skłodowska-Curie ITN program, where she studied how the extracellular matrix changes during lung cancer progression. During her postdoctoral fellowship at ETH Zurich, she studied how mechanical forces impact cardiovascular ageing and disease. Maria has led and executed preclinical projects, gaining experience in collaborations, protocol development, and trial design, which she aims to apply in medical research and innovation.

Luca Anceschi, Student - M.Sc. Biomedical Engineering, ETH Zürich
ML Engineer Intern
Luca is currently completing his Master's degree in Biomedical Engineering at ETH Zürich with focus on the Bio-imaging field, following a Bachelor's in Biomedical Engineering at Politecnico di Milano. At ETH he carried out projects with prof. Ralph Müller in the Laboratory for Bone Biomechanics and prof. Carlo Menon in the Biomedical and Mobile Health Technology Laboratory. He is passionate about leveraging novel machine and deep learning frameworks to analyze medical data.
.webp)
Sina Werner, B.A., University of St. Gallen (HSG)
Growth and Sales Associate
Sina Werner recently completed her Bachelor's degree in Business Administration at the University of St. Gallen (HSG). During her studies, she developed a strong interest in how social issues intersect with the business world. For her thesis, she explored how endometriosis and painful periods can impact women’s experiences and performance at work, aiming to bring more visibility to a topic that is often overlooked in professional settings. She is now beginning her Master’s in Innovation and Entrepreneurship at ZHAW, where she is excited to dive deeper into creative problem-solving and explore how innovative thinking can help build more inclusive and forward-thinking workplaces.
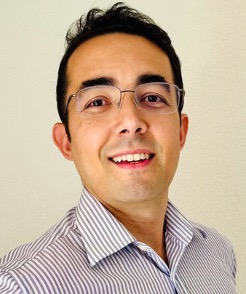
Cristovao Vieira, Ph.D.
Regulatory and Quality Assurance Specialist
Dr. Cristóvão Vieira holds a Master’s degree in Biotechnology from the University of Nottingham in the UK, obtained through the prestigious British Chevening Scholarship program. He was also awarded a Swiss Government Excellence Scholarship for his PhD, which focused on understanding cell communication during organ growth in plants at the University of Neuchâtel.
Dr. Cristóvão Vieira has also worked in regulatory and quality assurance activities in the chemical sector, combining academic and industry expertise into a unique professional profile.
Co-Founders and advisors
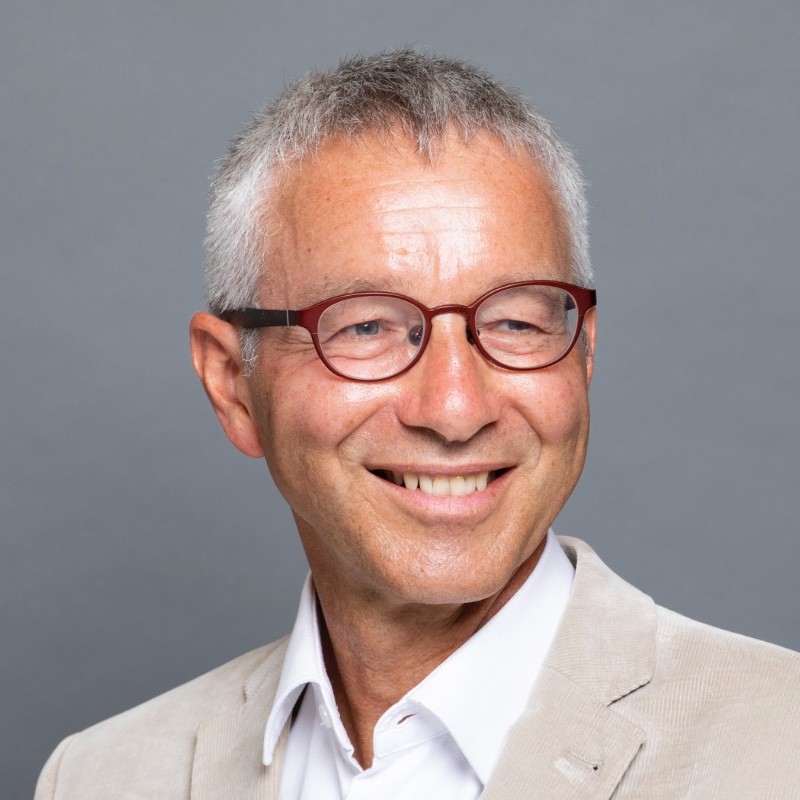
Joachim M. Buhmann, PhD, Prof.
Co-Founder, Research Advisory
Joachim M. Buhmann is full professor in Computer Science at ETH Zurich, where he leads the Information Science and Engineering group. His research interests cover the area of pattern recognition and data analysis, i.e., machine learning, statistical learning theory and applied statistics with focus in bioinformatics.
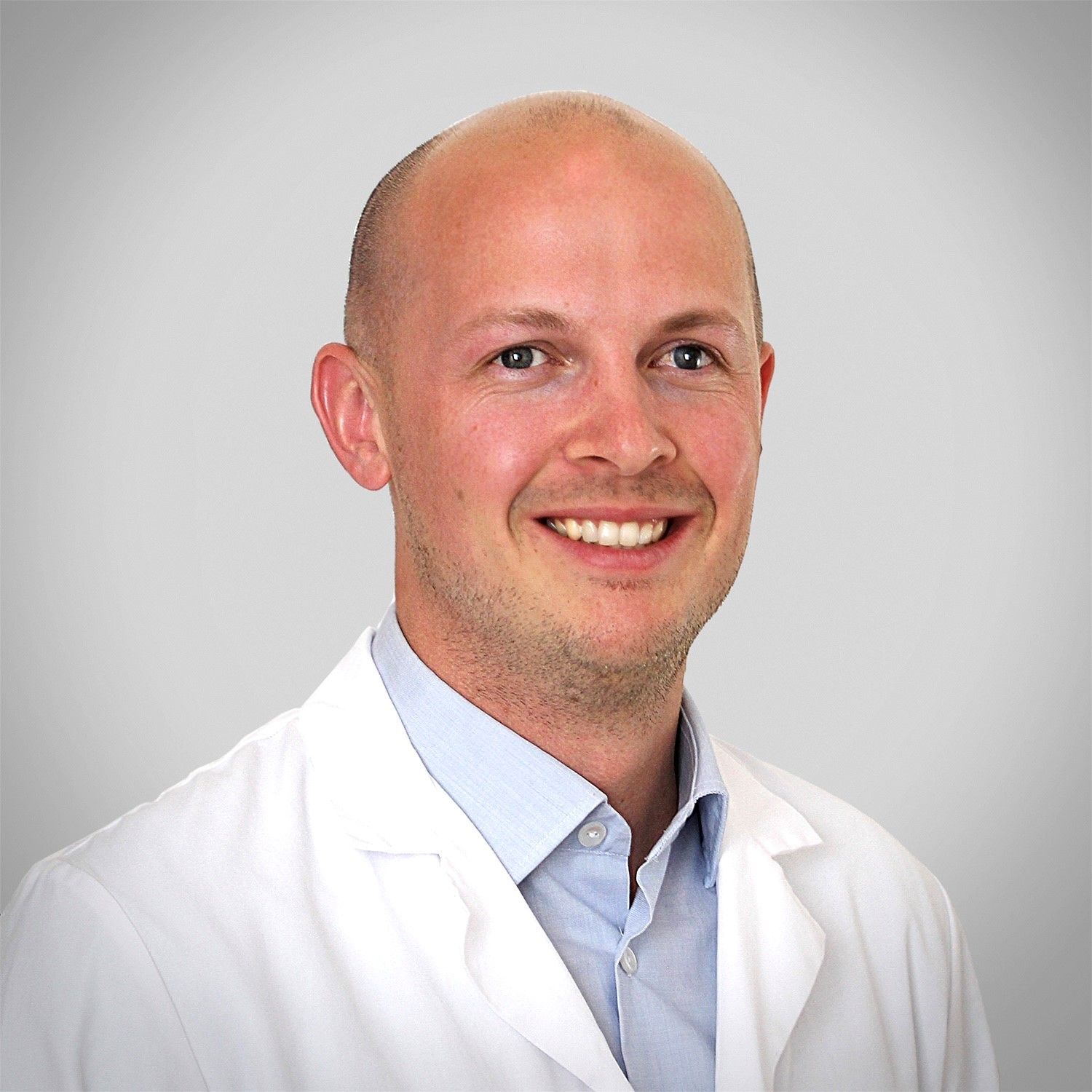
Julian Metzler, M.D.
Co-Founder, Medical Advisory
Julian M. Metzler is a consultant gynecologist at the University Hospital Zurich where he co-leads the Endometriosis center. His main focus is gynecological sonography and minimally-invasive gynecological surgery.

Julia Vogt, PhD, Prof.
Research Advisory
Julia Vogt is an assistant professor in Computer Science at ETH Zurich, where she leads the Medical Data Science Group. The focus of her research is on linking computer science with medicine, with the ultimate aim of personalized patient treatment.

Ece Özkan Elsen, PhD
Scientific Advisor
Ece Özkan Elsen is an established researcher in Computer Science at ETH Zurich. The focus of her research lie in enhancing the generalization, explainability, and fairness of machine learning models to address medical challenges and interpret medical data.